Low-Power Computer Vision
2021 ICCV Workshop (Virtual)
PROGRAM
http://iccv2021.thecvf.com/home
Preliminary Program for the Workshop
Time Zone: US Eastern Time (GMT - 4)
Time | Speaker | Event Title |
---|---|---|
8:00 | Orgnizers | Welcome |
8:05 | Winners present solutions of 2021 IEEE low-power computer vision challenge Moderator: Callie Hao, Georgia Tech
| |
8:35 | Selected Papers
| |
9:35 | Break | |
Invited Speeches, Moderator: Kate Saenko, Associate Professor, Boston University | ||
9:40 | Forrest Iandola, Co-founder and CEO, DeepScale (acquired by Tesla in 2019) | What have we actually accomplished in five years of efficient neural network research? |
10:00 | Kristen Grauman, Professor, University of Texas | Audio for efficient vision |
10:20 | Hee-Jun Park, Qualcomm AI SOC architect | Low Power Vision Processing with Dynamic Neural Networks |
10:40 | Carole-Jean Wu, Research Scientist, Facebook AI | Understanding and optimizing machine learning for the edge |
11:00 | Panel: Challenges and directions of low-power computer vision Moderator: Yiran Chen, Duke University Panelists:
| |
12:00 | Adjourn |
Biographies
Yiran Chen
Professor, Duke University
Yiran Chen received B.S (1998) and M.S. (2001) from Tsinghua University and Ph.D. (2005) from Purdue University. After five years in industry, he joined University of Pittsburgh in 2010 as Assistant Professor and then was promoted to Associate Professor with tenure in 2014, holding Bicentennial Alumni Faculty Fellow. He is now the Professor of the Department of Electrical and Computer Engineering at Duke University and serving as the director of the NSF AI Institute for Edge Computing Leveraging the Next-generation Networks (Athena) and the NSF Industry–University Cooperative Research Center (IUCRC) for Alternative Sustainable and Intelligent Computing (ASIC), and the co-director of Duke Center for Computational Evolutionary Intelligence (CEI). His group focuses on the research of new memory and storage systems, machine learning and neuromorphic computing, and mobile computing systems. Dr. Chen has published 1 book and about 500 technical publications and has been granted 96 US patents. He has served as the associate editor of a dozen international academic transactions/journals and served on the technical and organization committees of more than 60 international conferences. He is now serving as the Editor-in-Chief of the IEEE Circuits and Systems Magazine. He received seven best paper awards, one best poster award, and fourteen best paper nominations from international conferences and workshops. He received many professional awards and is the distinguished lecturer of IEEE CEDA (2018-2021). He is a Fellow of the ACM and IEEE and now serves as the chair of ACM SIGDA.
Adam Fuks
NXP Semiconductors
Adam Fuks is an Architecture Fellow at NXP Semiconductors in San Jose, California. He has over 20 years of experience in driving low-power IC design and architecture for a wide variety of products, including Application Processors for Smartphones, Microcontrollers and others.
Adam’s work sits at the intersection of several disciplines:
- Computer hardware architecture
- Real time Software architecture
- Digital Signal Processing algorithms and acceleration
- Machine Learning algorithms and acceleration
- Low Power architectures
Kristen Grauman
Professor, University of Texas
Kristen Grauman is a Professor in the Department of Computer Science at the University of Texas at Austin and a Research Scientist in Facebook AI Research (FAIR). Her research in computer vision and machine learning focuses on visual recognition, video, and embodied perception. Before joining UT-Austin in 2007, she received her Ph.D. at MIT. She is an IEEE Fellow, AAAI Fellow, Sloan Fellow, and recipient of the 2013 Computers and Thought Award. She and her collaborators have been recognized with several Best Paper awards in computer vision, including a 2011 Marr Prize and a 2017 Helmholtz Prize (test of time award). She served as an Associate Editor-in-Chief for PAMI and a Program Chair of CVPR 2015 and NeurIPS 2018.
Song Han
MIT
Song Han is an assistant professor at MIT’s EECS. He received his PhD degree from Stanford University. His research focuses on efficient deep learning computing. He proposed “deep compression” technique that can reduce neural network size by an order of magnitude without losing accuracy, and the hardware implementation “efficient inference engine” that first exploited pruning and weight sparsity in deep learning accelerators. His team’s work on hardware-aware neural architecture search that bring deep learning to IoT devices was highlighted by MIT News, Wired, Qualcomm News, VentureBeat, IEEE Spectrum, integrated in PyTorch and AutoGluon, and received many low-power computer vision contest awards in flagship AI conferences (CVPR’19, ICCV’19 and NeurIPS’19). Song received Best Paper awards at ICLR’16 and FPGA’17, Amazon Machine Learning Research Award, SONY Faculty Award, Facebook Faculty Award, NVIDIA Academic Partnership Award. Song was named “35 Innovators Under 35” by MIT Technology Review for his contribution on “deep compression” technique that “lets powerful artificial intelligence (AI) programs run more efficiently on low-power mobile devices.” Song received the NSF CAREER Award for “efficient algorithms and hardware for accelerated machine learning” and the IEEE “AIs 10 to Watch: The Future of AI” award.
Forrest Iandola
Co-founder and CEO, DeepScale (acquired by Tesla in 2019)
Forrest Iandola completed a PhD in EECS at UC Berkeley, where his research focused on deep neural networks. His best-known work includes deep learning infrastructure such as FireCaffe and efficient deep neural network models such as SqueezeNet and SqueezeDet. His research in deep learning led to the founding of DeepScale, which was acquired by Tesla in 2019.
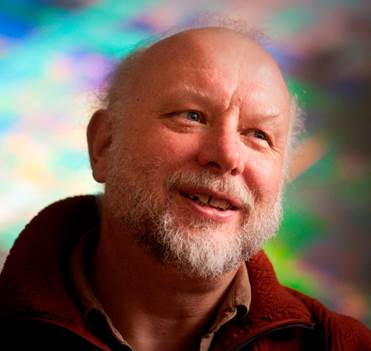
Kurt Keutzer
Professor, University of California, Berkeley
Kurt received his Ph.D. degree in Computer Science from Indiana University in 1984 and then joined the research division of AT&T Bell Laboratories. In 1991 he joined Synopsys, Inc. where he ultimately became Chief Technical Officer and Senior Vice-President of Research. In 1998 Kurt became Professor of Electrical Engineering and Computer Science at the University of California at Berkeley. Kurt’s research group is currently focused on using parallelism to accelerate the training and deployment of Deep Neural Networks for applications in computer vision, speech recognition, multi-media analysis, and computational finance. Kurt has published six books, over 250 refereed articles, and is among the most highly cited authors in Hardware and Design Automation. Kurt was elected a Fellow of the IEEE in 1996.
Hee-jun Park
Automotive System-on-Chip Architect, Qualcomm
Hee-jun Park works on modeling, simulation, and optimization of automotive SOCs for ADAS, Infotainment, and Telematics at Qualcomm. He has 201 granted patents in energy efficient processing in SOC hardware and software architectures across automotive, mobile phones, virtual/augmented/mixed reality, personal computers, and servers. He received his PhD degree from KAIST (Korea Advanced Institute of Science and Technology)
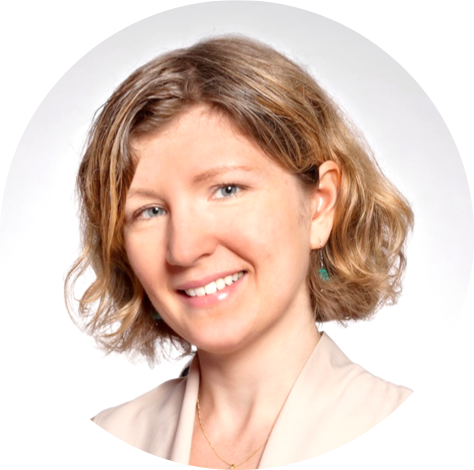
Kate Saenko
Boston University, saenko@bu.edu
Kate is an Associate Professor of Computer Science at Boston University and a consulting professor for the MIT-IBM Watson AI Lab. She leads the Computer Vision and Learning Group at BU, is the founder and co-director of the Artificial Intelligence Research (AIR) initiative, and member of the Image and Video Computing research group. Kate received a PhD from MIT and did her postdoctoral training at UC Berkeley and Harvard. Her research interests are in the broad area of Artificial Intelligence with a focus on dataset bias, adaptive machine learning, learning for image and language understanding, and deep learning.
Carole-Jean Wu
Research Scientist, Facebook AI Research
Carole-Jean Wu is a Research Scientist at Facebook AI Research. Her research lies in the domain of computer architecture. Her work has included designing energy- and memory-efficient systems, building and optimizing systems for machine learning execution at-scale. She is passionate about tackling system challenges to enable efficient, responsible AI execution. Carole-Jean chairs the MLPerf Recommendation Benchmark Advisory Board, co-chaired MLPerf Inference, and serves on the MLCommons Board as a director. Carole-Jean received her M.A. and Ph.D. from Princeton and B.Sc. from Cornell.
Organizers (ordered alphabetically by last names)
Name | Affiliation | Contact Info |
---|---|---|
Ming-Ching Chang | University at Albany | mchang2@albany.edu |
Shu-Ching Chen | Florida International University | chens@cs.fiu.edu |
Yiran Chen | Duke University | yiran.chen@duke.edu |
Callie Hao | Georgia Institute of Technology | callie.hao@gatech.edu |
Mark Liao | Institute of Information Science Academia Sinica | liao@iis.sinica.edu.tw |
(contact) Yung-Hsiang Lu | Purdue University | yunglu@purdue.edu |
Naveen Purushotham | Xilinx | npurusho@xilinx.com |
Mei-Ling Shyu | University of Miami | shyu@miami.edu |
Joseph Spisak | jspisak@fb.com | |
George K Thiruvathukal | Loyola University Chicago | gthiruvathukal@luc.edu |
Xuefeng Xiao | ByteDance Inc | xiaoxuefeng.ailab@bytedance.com |
Wei Zakharov | Purdue University | wzakharov@purdue.edu |
Paper Abstracts
- Knowledge Distillation for Low-Power Object Detection: A Simple Technique and Its Extensions for Training Compact Models Using Unlabeled Data
- Exploring the power of lightweight YOLOv4
- FOX-NAS: Fast, On-device and Explainable Neural Architecture Search
- Post-training deep neural network pruning via layer-wise calibration
Author: Amin Banitalebi-Dehkordi (Huawei Technologies Canada Co., Ltd.)
Abstract: The existing solutions for object detection distillation rely on the availability of both a teacher model and ground-truth labels. We propose a new perspective to relax this constraint. In our framework, a student is first trained with pseudo labels generated by the teacher, and then fine-tuned using labeled data, if any available. Extensive experiments demonstrate improvements over existing object detection distillation algorithms. In addition, decoupling the teacher and ground-truth distillation in this framework provides interesting properties such as: 1) using unlabeled data to further improve the student’s performance, 2) combining multiple teacher models of different architectures, even with different object categories, and 3) reducing the need for labeled data (with only 20% of COCO labels, this method achieves the same performance as the model trained on the entire set of labels). Furthermore, a by-product of this approach is the potential usage for domain adaptation. We verify these properties through extensive experiments.
Biography: Dr. Amin Banitalebi-Dehkordi - received his BASc and MASc degrees in electrical and computer engineering from the University of Tehran, Iran, in 2008 and 2011, and his PhD in the Digital Media Lab at the University of British Columbia (UBC), Canada, in 2014. His academic career has resulted in many publications in the fields of computer vision and pattern recognition, visual attention modeling, video quality assessment, and high dynamic range video. His industrial experience expands to areas in machine learning, deep learning, computer vision, NLP, and signal/image/video processing. Amin is currently a principal researcher in machine learning and technical lead at Vancouver Research Centre, Huawei Technologies Canada Co., Ltd.
Author: Chien-Yao Wang (Institute of Information Science, Academia Sinica)*; Hong-Yuan Mark Liao (Institute of Information Science, Academia Sinica, Taiwan); I-Hau Yeh (Elan Microelectronics Corporation); Yung-Yu Chuang (National Taiwan University); Youn-Long Lin (National Tsing Hua University)
Abstract: Research on deep learning has always had two main streams: (1) design a powerful network architecture and train it with existing learning methods to achieve the best results, and (2) design better learning methods so that the existing network architecture can achieve the best capability after training. In recent years, because mobile device has become popular, the requirement of low power consumption becomes a must. Under the requirement of low power consumption, we hope to design low-cost lightweight networks that can be effectively deployed at the edge, while it must have enough resources to be used and the inference speed must be fast enough. In this work, we set a very ambitious goal of exploring the power of lightweight neural networks. We utilize the analysis of data space, model's representational capacity, and knowledge projection space to construct an automated machine learning pipeline. Through this mechanism, we systematically derive the most suitable knowledge projection space between the data and the model. Our method can indeed automatically find learning strategies suitable for the target model and target application through exploration. Experiment results show that the proposed method can significantly enhance the accuracy of lightweight neural networks for object detection. We directly apply the lightweight model trained by our proposed method to a Jetson Xavier NX embedded module and a Kneron KL720 edge AI SoC as system solutions.
Biography: Chien-Yao Wang is currently a postdoctoral fellow with the Institute of Information Science, Academia Sinica, Taiwan. He received the B.S. degree in computer science and information engineering from National Central University, Zhongli, Taiwan, in 2013, and he received the Ph.D. degree from National Central University, Zhongli, Taiwan, in 2017. His research interests include signal processing, deep learning, and machine learning. He is an honorary member of Phi Tau Phi Scholastic Honor Society.
Author: Chia-Hsiang Liu (National Yang Ming Chiao Tung University)*; Yu-Shin Han (National Yang Ming Chiao Tung University ); Yuan-Yao Sung (National Yang Ming Chiao Tung University ); Yi Lee (National Yang Ming Chiao Tung University ); Hung-Yueh Chiang (The University of Texas at Austin); Kai-Chiang Wu (National Chiao Tung University)
Abstract: Neural architecture search can discover neural networks with good performance, and One-Shot approaches are prevalent. One-Shot approaches typically require a supernet with weight sharing and predictors that predict the performance of architecture. However, the previous methods take much time to generate performance predictors thus are inefficient. To this end, we propose FOX-NAS that consists of fast and explainable predictors based on simulated annealing and multivariate regression. Our method is quantization-friendly and can be efficiently deployed to the edge. The experiments on different hardware show that FOX-NAS models outperform some other popular neural network architectures. For example, FOX-NAS matches MobileNetV2 and EfficientNet-Lite0 accuracy with 240% and 40% less latency on the edge CPU. FOX-NAS is the 3rd place winner of the 2020 IEEE Low-Power Computer Vision Challenge (LPCVC), DSP classification track. See all evaluation results at https://lpcv.ai/competitions/2020. Search code and pre-trained models are released at https://github.com/great8nctu/FOX-NAS.
Biography: Chia-Hsiang Liu received a bachelor's degree in Electrical Engineering from National Chi Nan University, Taiwan and a Master's degree in Data Science and Engineering from National Yang Ming Chiao Tung University, Taiwan. During his study in the institute, he participated in the Semiconductor Moonshot Project of Taiwan's Ministry of Science and Technology. He won third place in the 2020 LPCV challenge. His research interests include: Deep learning and machine learning algorithm, Model optimization and deployment on edge devices, Data analysis.
Author: Ivan Lazarevich (Intel Corporation); Alexander Mr Kozlov (Intel Corp); Nikita Malinin (Intel Corporation)
Abstract: We present a post-training weight pruning method for deep neural networks that achieves accuracy levels tolerable for the production setting and that is sufficiently fast to be run on commodity hardware such as desktop CPUs or edge devices. We propose a data-free extension of the approach for computer vision models based on automatically-generated synthetic fractal images. We obtain state-of-the-art results for data-free neural network pruning, with ~1.5% top@1 accuracy drop for a ResNet50 on ImageNet at 50% sparsity rate. When using real data, we are able to get a ResNet50 model on ImageNet with 65% sparsity rate in 8-bit precision in a post-training setting with a ~1% top@1 accuracy drop. We release the code as a part of the OpenVINO(TM) Post-Training Optimization tool.
Biography: Ivan Lazarevich is a Senior Deep Learning R&D Engineer at Intel working on neural network compression. He has previously worked as a software engineer in computational physics at Intel. Ivan holds a Master's degree in Physics and has studied for a doctorate in computational neuroscience at Ecole normale superieure. Ivan's interests include deep neural network compression, computer vision, and application of machine learning to neuroscience.
Call For Papers
Computer vision technologies have made impressive progress in recent years, but often at the expenses of increasingly complex models needing more computational and storage resources. This workshop aims to improve the energy efficiency of computer vision solutions for running on systems with stringent resources, for example, mobile phones, drones, or renewable energy systems. Efficient computer vision can enable many new applications (e.g., wildlife observation) powered by ambient renewable energy (e.g., solar, vibration, and wind). This workshop will discuss the state of the art of low-power computer vision, challenges in creating efficient vision solutions, promising technologies that can achieve the goals, methods to acquire and label data, benchmarks and metrics to evaluate progress and success. Authors are encouraged to present innovation in any part of the entire systems, such as new hardware components, new algorithms, new methods for system integration, new semiconductor devices, and new computing paradigms. Authors are encouraged to discuss the following issues in their papers: the solution, the data for evaluation, and integration of the system, metrics for evaluation, and comparison with the state-of-the-art. This workshop emphasizes “system-level” solutions with implementations for demonstrations and experiments. Conceptual designs or solutions for individual components without integration into functional systems are discouraged.
Submissions
All submissions must be in the PDF format. Papers are limited to eight pages, including figures and tables, in the ICCV style. Additional pages containing only cited references are allowed.
- Template: http://iccv2021.thecvf.com/node/4#submission-guidelines
- Submission site: https://cmt3.research.microsoft.com/LPCVW2021
Important Dates:
- 2021/07/03 Title and abstract submission
- 2021/07/10 Paper submission
- 2021/07/31 Notifications and Reviews
- 2021/08/10 Final version (this date is set by the conference and cannot be extended)
- 2021/10/11 Workshop
Sponsors
Organizations interested in sponsoring the workshop, please contact Dr. Yung-Hsiang Lu, yunglu@purdue.edu. Check all sponsors at homepage Sponsors